It’s not every day that you stumble upon a book that completely shifts your perspective on your day-to-day work. That’s exactly what happened when I read “Data Mesh: Delivering Data-Driven Value at Scale” by Zhamak Dehghani. While I’ve spent years navigating data analytics, BI tools, and cloud-based platforms, this book offered a lens through which I began to rethink not just technical aspects of data, but the organizational and cultural structures surrounding it. The concept of Data Mesh isn’t just a technical framework, it’s a new way of looking at how data flows, how it’s managed, and most importantly, how it creates value.
Data mesh is a decentralized sociotechnical approach to share, access and manage analytical data in complex and large-scale environments – within or across organizations.
Zhamak Dehgani
Why Data Mesh Is a Game Changer
At its core, Data Mesh represents a fundamental shift in how organizations think about and handle data. For decades, companies have relied on centralized data platforms, data warehouses or data lakes to gather and store vast amounts of information. While these systems have served their purpose, they’ve also introduced significant challenges:
- Bottlenecks in data delivery
- Lack of domain expertise in data modeling
- A disconnect between data producers and consumers
To name a few… The list goes on.
If you’ve ever worked with data in a large corporation, you know exactly what I’m talking about. The vision of Data Mesh challenges this traditional model by distributing responsibility for data across domains. Each domain, whether it’s marketing, sales, finance, or operations, takes ownership of its data. These domains are responsible not only for producing and maintaining high-quality data but also for making it available and consumable by others. This principle, Domain-Oriented Data Ownership, forms the backbone of the Data Mesh approach.
This isn’t just about splitting up data responsibilities, it’s about empowering teams. When domains take ownership, they don’t just produce data – they create data products. These products aren’t raw datasets dumped into a central repository. Instead, they are polished, reliable, and reusable assets designed with clear documentation, defined access rules, and a focus on user needs. Semantics close to the business, by the business.
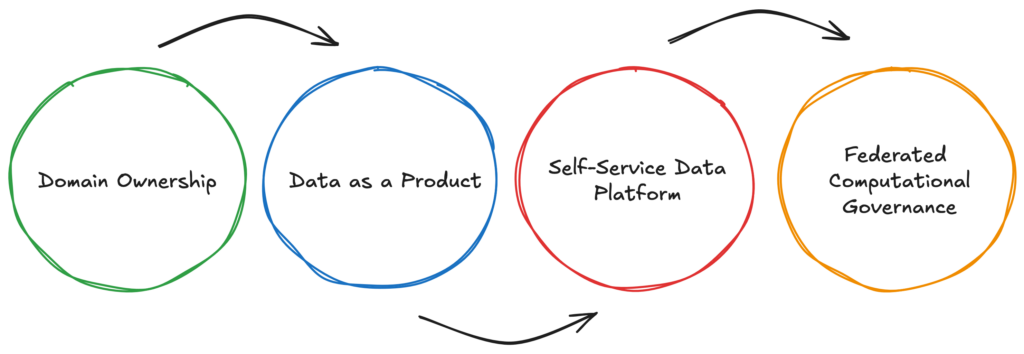
Data as a Product
The idea of treating data as a product is deceptively simple but incredibly powerful. Too often, data is viewed as an incidental byproduct of business processes – a raw resource waiting to be refined. But in the Data Mesh model, data is seen as a standalone product with its own lifecycle, from creation and QA to consumption and continuous improvement.
Think about a software product. It doesn’t just exist, it’s built, documented, marketed, and supported. Data products follow the same philosophy. They need to be discoverable, understandable, and reliable. They should be built with end-users in mind, whether those users are data scientists, analysts, or other domains consuming that data.
This shift in mindset also creates accountability. When teams know their data is going to be used by others, they’re more likely to ensure its quality, maintain its integrity, and keep it up to date. Data stops being an afterthought and becomes an essential deliverable.
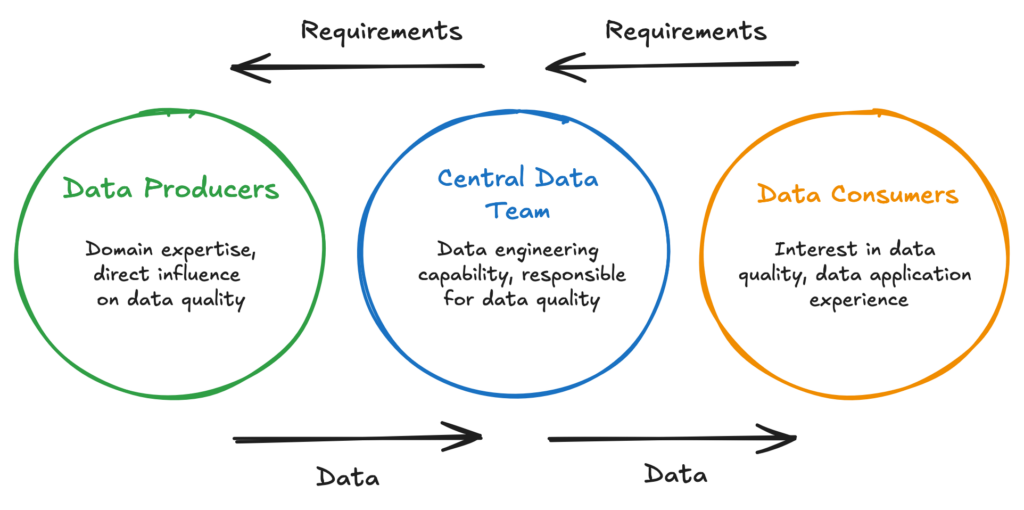
Self-Serve Data Platforms
A critical enabler of the Data Mesh philosophy is the self-serve data platform. Traditional data architectures often create bottlenecks because every data request must go through a central IT or data team. This approach is slow, inefficient, and frustrating for both technical and business teams.
A self-serve data platform changes this dynamic. It provides domain teams with the tools and capabilities they need to handle their data independently – whether it’s ingesting new datasets, transforming them into valuable insights, or securely sharing them with others. These platforms aren’t just about technology, they represent a cultural shift. Teams need to trust the platform, understand how to use it effectively, and feel empowered to make decisions about their data without waiting for approvals from a centralized authority.
However, building a self-serve platform isn’t simply about giving everyone access to the same tools. It requires careful design, user-centric interfaces, robust governance frameworks, and ongoing support to ensure domains can truly operate independently.
Federated Computational Governance
At first glance, decentralizing data ownership and empowering teams might sound like a recipe for chaos. Without oversight, teams could end up creating inconsistent data products, violating compliance standards, or duplicating efforts- or like I tend to call it, data anarchy. That’s where federated computational governance comes in.
Federated governance isn’t about creating rigid rules enforced by a central authority. Instead, it’s about creating a set of global standards and allowing domains to apply those standards in a way that makes sense for their specific needs. Think of it like a city’s building codes – every building follows core safety standards, but the architects have flexibility in design.
This balance between autonomy and standardization is what keeps a Data Mesh functioning effectively. Domains have the freedom to innovate, but they’re still accountable for meeting quality, security, and interoperability requirements.
Moving Beyond Centralized Architectures
Centralized data architectures aren’t inherently bad, they’ve served many organizations well for years. But as data volumes grow, use cases diversify, and teams become more specialized, the limitations of centralization become clear. Centralized systems struggle with scalability, responsiveness, and adaptability.
Data Mesh doesn’t eliminate the need for central infrastructure entirely. Instead, it reimagines how that infrastructure supports decentralized teams. The result is an architecture that’s both flexible and scalable, capable of adapting to new challenges without requiring constant reengineering.
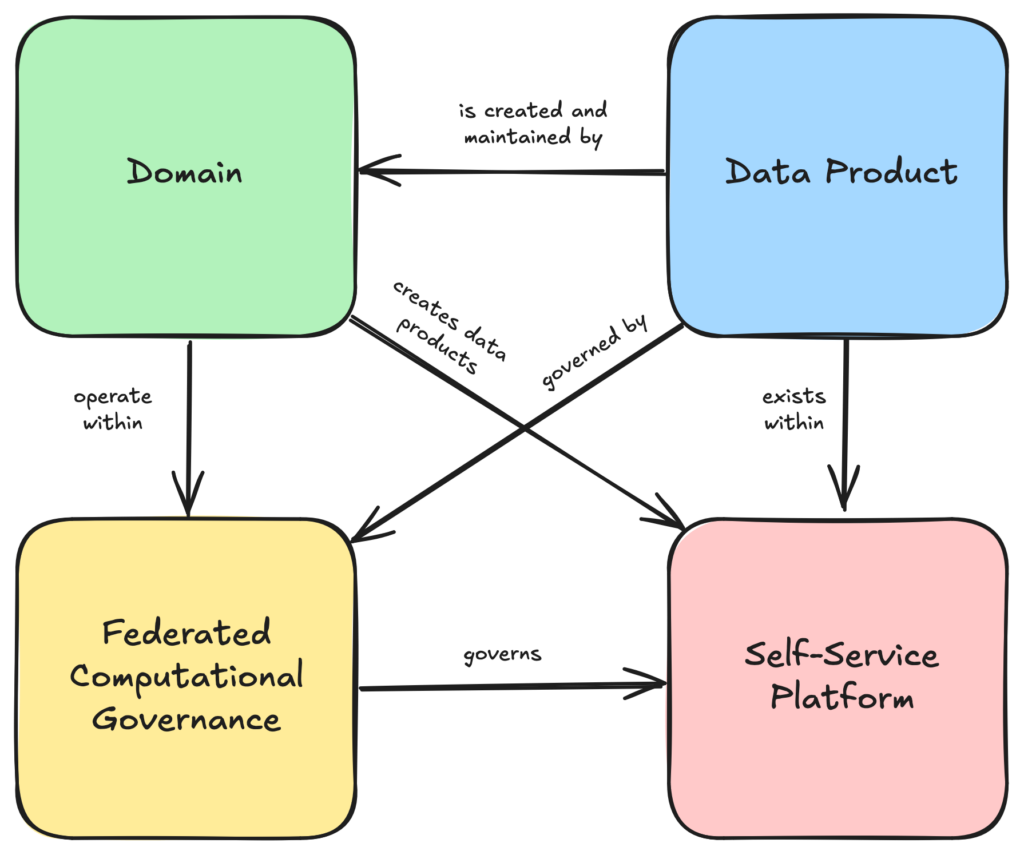
Hardcore or Hybrid?
While the vision of Data Mesh shines brightly on paper, its real-world implementation often proves far more challenging. The decentralized approach of domain-driven ownership and data-as-a-product can create fragmented silos, inconsistent standards, and governance headaches if executed without a strong backbone. Many organizations attempting a “pure” Data Mesh end up overwhelmed by the operational complexity, lack of technical maturity across domains, and an inconsistent understanding of data accountability, creating even more frustration than value.
A more pragmatic approach is often a hybrid model: maintaining a centralized data platform team that builds and governs a robust self-serve platform while enabling domains to create and manage their data products within a well-defined framework. This approach preserves the scalability and flexibility of Data Mesh principles without losing the efficiencies of centralization.
In practice, the central team focuses on enabling domains with shared tools, security standards, and cost governance, while individual departments leverage these resources to build their own data products. This balance ensures that while domains have the autonomy to innovate, they remain aligned with a cohesive, enterprise-wide data strategy.
So, Is Data Mesh the New Gold Standard?
Data Mesh isn’t just another architectural trend – it’s a profound rethinking of how we manage, share, and extract value from data. Its principles – domain ownership, data as a product, self-serve platforms, and federated governance aren’t isolated ideas. They work together to create a cohesive and resilient data ecosystem.
Implementing Data Mesh isn’t easy, and it isn’t a one-size-fits-all solution. But for organizations willing to embrace its principles, the rewards are significant: faster access to data, improved scalability, and a culture where data truly drives decisions.
If you’ve explored Data Mesh principles or have thoughts on how they can be implemented effectively, I’d love to hear from you.